Artificial intelligence (AI) has been a transformative force in various industries, revolutionizing operations, improving efficiency, and driving innovation. The AI upgrade cycle is an essential aspect of the evolution and progression of AI technologies. Through a continuous process of development, enhancement, and advancement, AI systems are constantly being refined to push the boundaries of what is possible.
The AI upgrade cycle encompasses various stages that are essential in the evolution of AI technologies. At the core of this cycle is the concept of continuous learning and evolution. AI systems are designed to adapt and improve based on user interactions, data input, and feedback. This adaptive nature allows AI systems to become more efficient and effective over time.
One crucial stage in the AI upgrade cycle is data gathering and processing. Data is the lifeblood of AI systems, providing the necessary input for algorithms to learn and make informed decisions. Through the collection and processing of vast amounts of data, AI systems can improve their accuracy and performance.
Another important stage in the AI upgrade cycle is algorithm development and optimization. Algorithms are the driving force behind AI systems, enabling them to perform tasks, make predictions, and automate processes. By continually refining and optimizing algorithms, AI systems can enhance their capabilities and deliver more accurate results.
Furthermore, model training and testing play a significant role in the AI upgrade cycle. AI models need to be trained on large datasets to learn patterns and relationships within the data. Through rigorous testing and validation processes, AI systems can ensure their reliability and accuracy in real-world applications.
Moreover, the deployment and integration of AI systems into existing infrastructure are crucial steps in the AI upgrade cycle. Seamless integration with existing systems and workflows is essential for maximizing the benefits of AI technologies. By effectively deploying AI systems, organizations can streamline operations, improve decision-making processes, and drive business growth.
Additionally, continuous monitoring and feedback mechanisms are essential components of the AI upgrade cycle. Monitoring AI systems in real-time allows for the identification of potential issues, performance bottlenecks, and areas for improvement. User feedback and input also play a vital role in guiding the evolution of AI technologies and ensuring that they meet user needs and expectations.
In conclusion, the AI upgrade cycle is a dynamic and iterative process that drives the evolution and advancement of AI technologies. By continuously learning, adapting, and improving, AI systems can push the boundaries of what is possible and deliver transformative impact across industries. Embracing the AI upgrade cycle is crucial for organizations looking to harness the full potential of AI technologies and stay at the forefront of innovation and competitiveness.
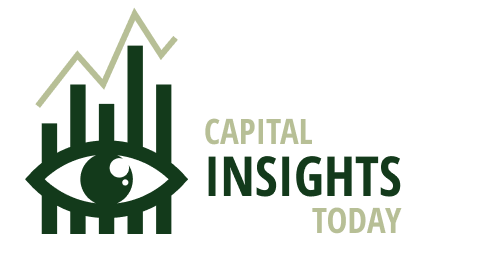