The potential dangers posed by artificial intelligence have long been a topic of debate and concern. In recent years, numerous advancements in AI technology have raised both excitement and anxiety about the future of these intelligent systems. One issue that is often overlooked but is of significant importance is AI’s hallucination problem.
Hallucinations in AI refer to situations where neural networks or algorithms generate outputs that deviate from reality or intended tasks. These hallucinations can potentially lead AI systems to make critical errors or produce malicious outcomes. Despite the potential risks associated with AI hallucinations, the issue has not received widespread attention compared to other AI ethics concerns.
One major factor contributing to AI hallucinations is the lack of comprehensive data and training sets. AI systems heavily rely on data to learn and make decisions. When these datasets are incomplete, biased, or corrupted, AI algorithms can produce inaccurate or hallucinatory results. Addressing this issue requires rigorous data collection, curation, and validation processes to ensure that AI models are trained on reliable and diverse datasets.
Another contributing factor to AI hallucinations is the complexity of neural networks and algorithms. As AI systems become more sophisticated and capable of handling complex tasks, they may also become more susceptible to generating hallucinatory outputs. Understanding the inner workings of AI algorithms and implementing robust validation mechanisms can help mitigate the risk of hallucinations.
Moreover, the black-box nature of AI systems poses a challenge in detecting and correcting hallucinations. Many AI algorithms operate as opaque systems, making it difficult for humans to interpret their decision-making processes. Enhancing transparency and interpretability in AI models can facilitate the identification and mitigation of hallucinations.
To address the AI hallucination problem effectively, a multidisciplinary approach involving experts in AI research, ethics, psychology, and policy is essential. Collaborative efforts can lead to the development of guidelines, standards, and frameworks for mitigating the risks associated with AI hallucinations. Additionally, fostering open dialogue and public awareness about the implications of AI hallucinations can help drive responsible AI development and deployment.
In conclusion, the issue of AI hallucinations is a complex and critical challenge that requires immediate attention and action. By understanding the underlying causes of AI hallucinations, promoting transparency and interpretability in AI systems, and fostering interdisciplinary collaboration, we can proactively address the risks and implications associated with AI hallucinations. Ignoring this problem could have far-reaching consequences for the future of AI technology and society as a whole. It is crucial to prioritize the ethical and responsible development of AI to ensure a safe and beneficial AI-driven future.
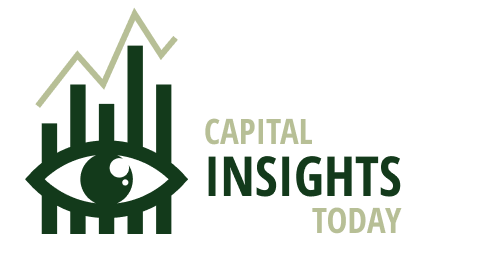